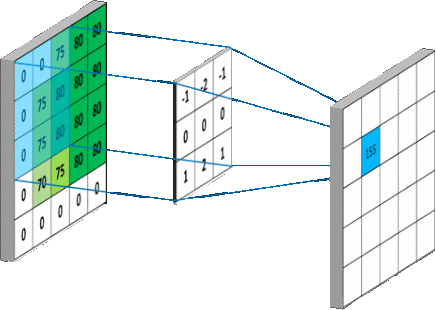
We are interested in studying the impact of applying various pre-processing methods on the detection efficiency of Viola-Jones detector. The pre-processing has to be blind so to be applied in all scenarios and still we do get better performance.
Explore the docs »
View Demo
·
Report Bug
·
Request Feature
Table of Contents
Here's a blank template to get started: To avoid retyping too much info. Do a search and replace with your text editor for the following: Bhartendu-Kumar
, DIP
, twitter_handle
, linkedin_username
, email
, email_client
, project_title
, project_description
This is an example of how you may give instructions on setting up your project locally. To get a local copy up and running follow these simple example steps.
This is an example of how to list things you need to use the software and how to install them.
- npm
npm install npm@latest -g
- Get a free API Key at https://example.com
- Clone the repo
git clone https://github.com/Bhartendu-Kumar/DIP.git
- Install NPM packages
npm install
- Enter your API in
config.js
const API_KEY = 'ENTER YOUR API';
Use this space to show useful examples of how a project can be used. Additional screenshots, code examples and demos work well in this space. You may also link to more resources.
For more examples, please refer to the Documentation
- [] Feature 1
- [] Feature 2
- [] Feature 3
- [] Nested Feature
See the open issues for a full list of proposed features (and known issues).
Contributions are what make the open source community such an amazing place to learn, inspire, and create. Any contributions you make are greatly appreciated.
If you have a suggestion that would make this better, please fork the repo and create a pull request. You can also simply open an issue with the tag "enhancement". Don't forget to give the project a star! Thanks again!
- Fork the Project
- Create your Feature Branch (
git checkout -b feature/AmazingFeature
) - Commit your Changes (
git commit -m 'Add some AmazingFeature'
) - Push to the Branch (
git push origin feature/AmazingFeature
) - Open a Pull Request
Distributed under the MIT License. See LICENSE.txt
for more information.
Your Name - @twitter_handle - email@email_client.com
Project Link: https://github.com/Bhartendu-Kumar/DIP
DIP project
DataSets:
- MIT CBCL
- BioID
- Yale
- Caltech
- SoF
- Orl
- Non - Face Dataset (Collected Heterogeneously)
Pre-Processing Methods Studied:
- Low Pass Filters:
- gaussian blur
- bilateral filter
- Deconvolutions
- Blind Deconvolution (richardson lucy)
- tv_chambolle
- BMD (partially implemented , not converging yet)
- Retinex
- SSR
- MSR
- retinex FM
- NMBM
- Normalization
- HOMO
- Histogram Manipulation
- CLAHE
- HE
- log intensity stretch
- full scale intensity stretch
The pipeline is as:
Step 1: Download the DataSets (from their owner sites) and use the scripts in the dataset_helper_scripts directory to convert all to greyscale and change extensions like (.gif and .pgm) to common extensions. Also arrange all images of a dataset in a single folder using these scripts.
Step 2: Arrange the data directory like shown has 2 subdirectories: faces non-faces These further have their particular sub-directories with name of the DATASET
----! Important, for illuastration "data" folder has the desired structure, just that it has few images
Step 3: Run all the "driver scripts" in the root directory of this repository. This will create an "output" directory with the result of all preprocessing methods.
Step 4: Use "csv_scripts" directory to get the performance metrics from the "output" directory
Step 5: "analysis" directory has the final performance csv files of each pre-procesing method
Step 6: Published report directory has the final findings in form of csv file.