Architecture | Results | Examples | Documentation
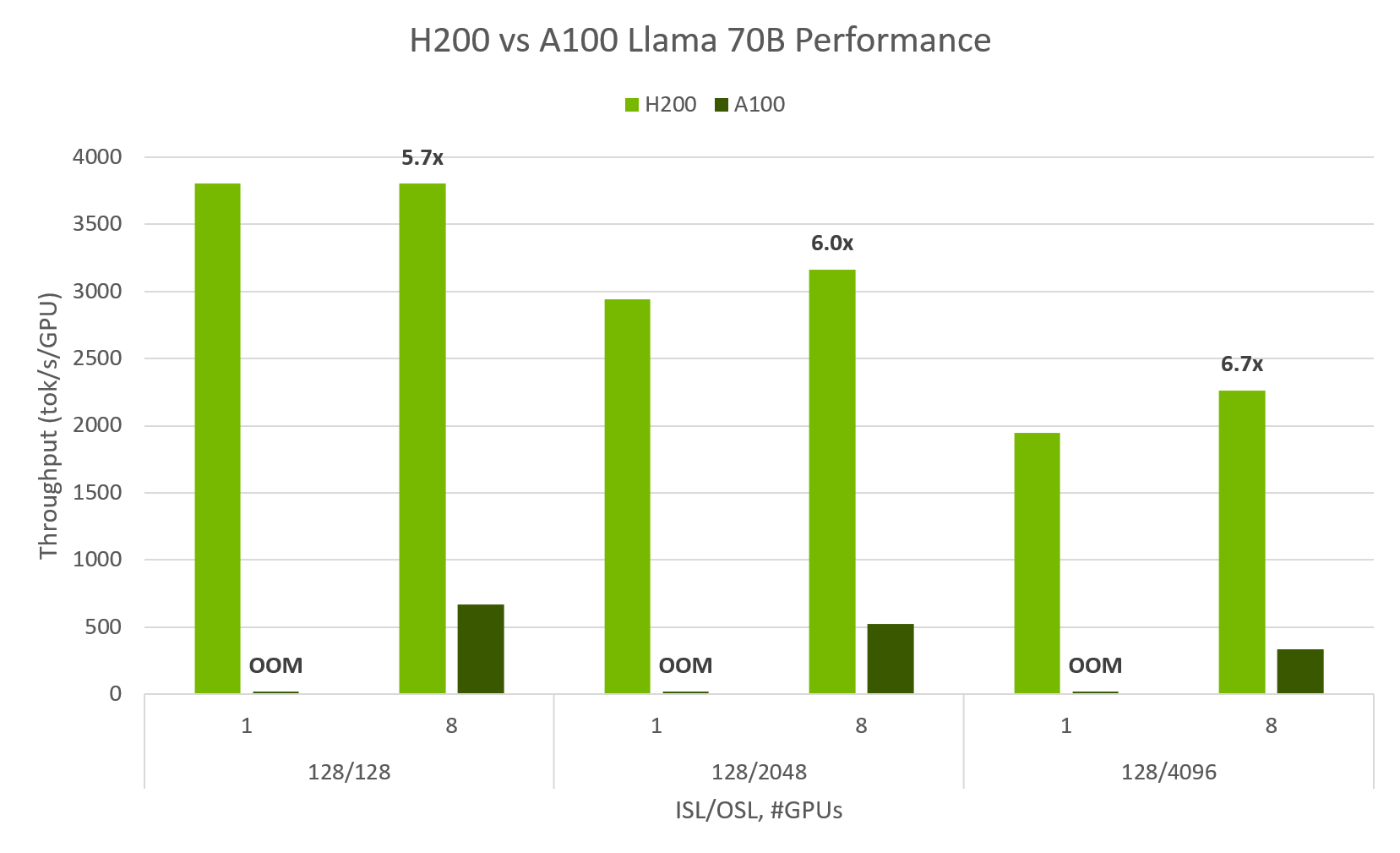
H200 with INT4 AWQ, runs Falcon-180B on a single GPU.
H200 is now 2.4x faster on Llama-70B with recent improvements to TensorRT-LLM GQA; up to 6.7x faster than A100.
- [2023/11/27] SageMaker LMI now supports TensorRT-LLM - improves throughput by 60%, compared to previous version
- [2023/11/13] H200 achieves nearly 12,000 tok/sec on Llama2-13B
- [2023/10/22] 🚀 RAG on Windows using TensorRT-LLM and LlamaIndex 🦙
- [2023/10/19] Getting Started Guide - Optimizing Inference on Large Language Models with NVIDIA TensorRT-LLM, Now Publicly Available
- [2023/10/17] Large Language Models up to 4x Faster on RTX With TensorRT-LLM for Windows
2023/11/27 - Amazon Sagemaker 2023/11/17 - Perplexity ; 2023/10/31 - Phind ; 2023/10/12 - Databricks (MosaicML) ; 2023/10/04 - Perplexity ; 2023/09/27 - CloudFlare;
- TensorRT-LLM Overview
- Installation
- Quick Start
- Support Matrix
- Performance
- Advanced Topics
- Troubleshooting
- Release notes
TensorRT-LLM provides users with an easy-to-use Python API to define Large Language Models (LLMs) and build TensorRT engines that contain state-of-the-art optimizations to perform inference efficiently on NVIDIA GPUs. TensorRT-LLM also contains components to create Python and C++ runtimes that execute those TensorRT engines. It also includes a backend for integration with the NVIDIA Triton Inference Server; a production-quality system to serve LLMs. Models built with TensorRT-LLM can be executed on a wide range of configurations going from a single GPU to multiple nodes with multiple GPUs (using Tensor Parallelism and/or Pipeline Parallelism).
The Python API of TensorRT-LLM is architectured to look similar to the
PyTorch API. It provides users with a
functional module containing functions like
einsum
, softmax
, matmul
or view
. The layers
module bundles useful building blocks to assemble LLMs; like an Attention
block, a MLP
or the entire Transformer
layer. Model-specific components,
like GPTAttention
or BertAttention
, can be found in the
models module.
TensorRT-LLM comes with several popular models pre-defined. They can easily be modified and extended to fit custom needs. See below for a list of supported models.
To maximize performance and reduce memory footprint, TensorRT-LLM allows the
models to be executed using different quantization modes (see
examples/gpt
for concrete examples). TensorRT-LLM supports
INT4 or INT8 weights (and FP16 activations; a.k.a. INT4/INT8 weight-only) as
well as a complete implementation of the
SmoothQuant technique.
For a more detailed presentation of the software architecture and the key concepts used in TensorRT-LLM, we recommend you to read the following document.
For Windows installation, see Windows
.
TensorRT-LLM must be built from source, instructions can be found here. An image of a Docker container with TensorRT-LLM and its Triton Inference Server Backend will be made available soon.
The remaining commands in that document must be executed from the TensorRT-LLM container.
To create a TensorRT engine for an existing model, there are 3 steps:
- Download pre-trained weights,
- Build a fully-optimized engine of the model,
- Deploy the engine.
The following sections show how to use TensorRT-LLM to run the BLOOM-560m model.
0. In the BLOOM folder
Inside the Docker container, you have to install the requirements:
pip install -r examples/bloom/requirements.txt
git lfs install
1. Download the model weights from HuggingFace
From the BLOOM example folder, you must download the weights of the model.
cd examples/bloom
rm -rf ./bloom/560M
mkdir -p ./bloom/560M && git clone https://huggingface.co/bigscience/bloom-560m ./bloom/560M
2. Build the engine
# Single GPU on BLOOM 560M
python build.py --model_dir ./bloom/560M/ \
--dtype float16 \
--use_gemm_plugin float16 \
--use_gpt_attention_plugin float16 \
--output_dir ./bloom/560M/trt_engines/fp16/1-gpu/
See the BLOOM example for more details and options regarding the build.py
script.
3. Run
The ../summarize.py
script can be used to perform the summarization of articles
from the CNN Daily dataset:
python ../summarize.py --test_trt_llm \
--hf_model_dir ./bloom/560M/ \
--data_type fp16 \
--engine_dir ./bloom/560M/trt_engines/fp16/1-gpu/
More details about the script and how to run the BLOOM model can be found in the example folder. Many more models than BLOOM are implemented in TensorRT-LLM. They can be found in the examples directory.
TensorRT-LLM optimizes the performance of a range of well-known models on NVIDIA GPUs. The following sections provide a list of supported GPU architectures as well as important features implemented in TensorRT-LLM.
TensorRT-LLM is rigorously tested on the following GPUs:
If a GPU is not listed above, it is important to note that TensorRT-LLM is expected to work on GPUs based on the Volta, Turing, Ampere, Hopper and Ada Lovelace architectures. Certain limitations may, however, apply.
Various numerical precisions are supported in TensorRT-LLM. The support for some of those numerical features require specific architectures:
FP32 | FP16 | BF16 | FP8 | INT8 | INT4 | |
---|---|---|---|---|---|---|
Volta (SM70) | Y | Y | N | N | Y | Y |
Turing (SM75) | Y | Y | N | N | Y | Y |
Ampere (SM80, SM86) | Y | Y | Y | N | Y | Y |
Ada-Lovelace (SM89) | Y | Y | Y | Y | Y | Y |
Hopper (SM90) | Y | Y | Y | Y | Y | Y |
In this release of TensorRT-LLM, the support for FP8 and quantized data types (INT8 or INT4) is not implemented for all the models. See the precision document and the examples folder for additional details.
TensorRT-LLM contains examples that implement the following features.
- Multi-head Attention(MHA)
- Multi-query Attention (MQA)
- Group-query Attention(GQA)
- In-flight Batching
- Paged KV Cache for the Attention
- Tensor Parallelism
- Pipeline Parallelism
- INT4/INT8 Weight-Only Quantization (W4A16 & W8A16)
- SmoothQuant
- GPTQ
- AWQ
- FP8
- Greedy-search
- Beam-search
- RoPE
In this release of TensorRT-LLM, some of the features are not enabled for all the models listed in the examples folder.
The list of supported models is:
- Baichuan
- Bert
- Blip2
- BLOOM
- ChatGLM
- Falcon
- Flan-T5
- GPT
- GPT-J
- GPT-Nemo
- GPT-NeoX
- InternLM
- LLaMA
- LLaMA-v2
- Mistral
- MPT
- mT5
- OPT
- Qwen
- Replit Code
- SantaCoder
- StarCoder
- T5
- Whisper
Note: Encoder-Decoder provides general encoder-decoder functionality that supports many encoder-decoder models such as T5 family, BART family, Whisper family, etc. We unroll the exact model names in the list above to let users find specific models easier.
Please refer to the performance page for performance numbers. That page contains measured numbers for four variants of popular models (GPT-J, LLAMA-7B, LLAMA-70B, Falcon-180B), measured on the H100, L40S and A100 GPU(s).
This document describes the different quantization methods implemented in TensorRT-LLM and contains a support matrix for the different models.
TensorRT-LLM supports in-flight batching of requests (also known as continuous batching or iteration-level batching). It's a technique that aims at reducing wait times in queues, eliminating the need for padding requests and allowing for higher GPU utilization.
TensorRT-LLM implements several variants of the Attention mechanism that appears in most the Large Language Models. This document summarizes those implementations and how they are optimized in TensorRT-LLM.
TensorRT-LLM uses a declarative approach to define neural networks and contains techniques to optimize the underlying graph. For more details, please refer to doc
TensorRT-LLM provides C++ and Python tools to perform benchmarking. Note, however, that it is recommended to use the C++ version.
-
It's recommended to add options
–shm-size=1g –ulimit memlock=-1
to the docker or nvidia-docker run command. Otherwise you may see NCCL errors when running multiple GPU inferences. See https://docs.nvidia.com/deeplearning/nccl/user-guide/docs/troubleshooting.html#errors for details. -
When building models, memory-related issues such as
[09/23/2023-03:13:00] [TRT] [E] 9: GPTLMHeadModel/layers/0/attention/qkv/PLUGIN_V2_Gemm_0: could not find any supported formats consistent with input/output data types
[09/23/2023-03:13:00] [TRT] [E] 9: [pluginV2Builder.cpp::reportPluginError::24] Error Code 9: Internal Error (GPTLMHeadModel/layers/0/attention/qkv/PLUGIN_V2_Gemm_0: could not find any supported formats consistent with input/output data types)
may happen. One possible solution is to reduce the amount of memory needed by
reducing the maximum batch size, input and output lengths. Another option is to
enable plugins, for example: --use_gpt_attention_plugin
.
- MPI + Slurm
TensorRT-LLM is a
MPI-aware package
that uses mpi4py
. If you are
running scripts in a Slurm environment, you might
encounter interferences:
--------------------------------------------------------------------------
PMI2_Init failed to initialize. Return code: 14
--------------------------------------------------------------------------
--------------------------------------------------------------------------
The application appears to have been direct launched using "srun",
but OMPI was not built with SLURM's PMI support and therefore cannot
execute. There are several options for building PMI support under
SLURM, depending upon the SLURM version you are using:
version 16.05 or later: you can use SLURM's PMIx support. This
requires that you configure and build SLURM --with-pmix.
Versions earlier than 16.05: you must use either SLURM's PMI-1 or
PMI-2 support. SLURM builds PMI-1 by default, or you can manually
install PMI-2. You must then build Open MPI using --with-pmi pointing
to the SLURM PMI library location.
Please configure as appropriate and try again.
--------------------------------------------------------------------------
As a rule of thumb, if you are running TensorRT-LLM interactively on a Slurm
node, prefix your commands with mpirun -n 1
to run TensorRT-LLM in a
dedicated MPI environment, not the one provided by your Slurm allocation.
For example: mpirun -n 1 python3 examples/gpt/build.py ...
- TensorRT-LLM requires TensorRT 9.2 and 23.10 containers.
-
Models
- ChatGLM3
- InternLM (contributed by @wangruohui)
- Mistral 7B (developed in collaboration with Mistral.AI)
- MQA/GQA support to MPT (and GPT) models (contributed by @bheilbrun)
- Qwen (contributed by @Tlntin and @zhaohb)
- Replit Code V-1.5 3B (external contribution)
- T5, mT5, Flan-T5 (Python runtime only)
-
Features
- Add runtime statistics related to active requests and KV cache utilization from the batch manager (see the batch manager documentation)
- Add
sequence_length
tensor to support proper lengths in beam-search (when beam-width > 1 - see tensorrt_llm/batch_manager/GptManager.h) - BF16 support for encoder-decoder models (Python runtime - see examples/enc_dec)
- Improvements to memory utilization (CPU and GPU - including memory leaks)
- Improved error reporting and memory consumption
- Improved support for stop and bad words
- INT8 SmoothQuant and INT8 KV Cache support for the Baichuan models (see examples/baichuan)
- INT4 AWQ Tensor Parallelism support and INT8 KV cache + AWQ/weight-only support for the GPT-J model (see examples/gptj)
- INT4 AWQ support for the Falcon models (see examples/falcon)
- LoRA support (functional preview only - limited to the Python runtime, only QKV support and not optimized in terms of runtime performance) for the GPT model (see the Run LoRA with the Nemo checkpoint in the GPT example)
- Multi-GPU support for encoder-decoder models (Python runtime - see examples/enc_dec)
- New heuristic for launching the Multi-block Masked MHA kernel (similar to FlashDecoding - see decoderMaskedMultiheadAttentionLaunch.h)
- Prompt-Tuning support for GPT and LLaMA models (see the Prompt-tuning Section in the GPT example)
- Performance optimizations in various CUDA kernels
- Possibility to exclude input tokens from the output (see
excludeInputInOutput
inGptManager
) - Python binding for the C++ runtime (GptSession - see
pybind
) - Support for different micro batch sizes for context and generation
phases with pipeline parallelism (see
GptSession::Config::ctxMicroBatchSize
andGptSession::Config::genMicroBatchSize
in tensorrt_llm/runtime/gptSession.h) - Support for "remove input padding" for encoder-decoder models (see examples/enc_dec)
- Support for context and generation logits (see
mComputeContextLogits
andmComputeGenerationLogits
in tensorrt_llm/runtime/gptModelConfig.h) - Support for
logProbs
andcumLogProbs
(see"output_log_probs"
and"cum_log_probs"
inGptManager
) - Update to CUTLASS 3.x
-
Bug fixes
- Fix for ChatGLM2 #93 and #138
- Fix tensor names error "RuntimeError: Tensor names
(
host_max_kv_cache_length
) in engine are not the same as expected in the main branch" #369 - Fix weights split issue in BLOOM when
world_size = 2
("array split does not result in an equal division") #374 - Fix SmoothQuant multi-GPU failure with tensor parallelism is 2 #267
- Fix a crash in GenerationSession if stream keyword argument is not None #202
- Fix a typo when calling PyNVML API [BUG] code bug #410
- Fix bugs related to the improper management of the
end_id
for various models [C++ and Python] - Fix memory leaks [C++ code and Python models]
- Fix the std::alloc error when running the gptManagerBenchmark -- issue gptManagerBenchmark std::bad_alloc error #66
- Fix a bug in pipeline parallelism when beam-width > 1
- Fix a bug with Llama GPTQ due to improper support of GQA
- Fix issue #88
- Fix an issue with the Huggingface Transformers version #16
- Fix link jump in windows readme.md #30 - by @yuanlehome
- Fix typo in batchScheduler.h #56 - by @eltociear
- Fix typo #58 - by @RichardScottOZ
- Fix Multi-block MMHA: Difference between
max_batch_size
in the engine builder andmax_num_sequences
in TrtGptModelOptionalParams? #65 - Fix the log message to be more accurate on KV cache #224
- Fix Windows release wheel installation: Failed to install the release wheel for Windows using pip #261
- Fix missing torch dependencies: [BUG] The batch_manage.a choice error in --cpp-only when torch's cxx_abi version is different with gcc #151
- Fix linking error during compiling google-test & benchmarks #277
- Fix logits dtype for Baichuan and ChatGLM: segmentation fault caused by the lack of bfloat16 #335
- Minor bug fixes
- TensorRT-LLM v0.5.0 is the first public release.
- The hang reported in issue #149 has not been reproduced by the TensorRT-LLM team. If it is caused by a bug in TensorRT-LLM, that bug may be present in that release
You can use GitHub issues to report issues with TensorRT-LLM.