Tools like TorchIO are a symptom of the maturation of medical AI research using deep learning techniques.
Jack Clark, Policy Director at OpenAI (link).
Package |
|
Docs |
|
Build |
|
Coverage |
|
Code |
|
Notebook |
|
Social |
|
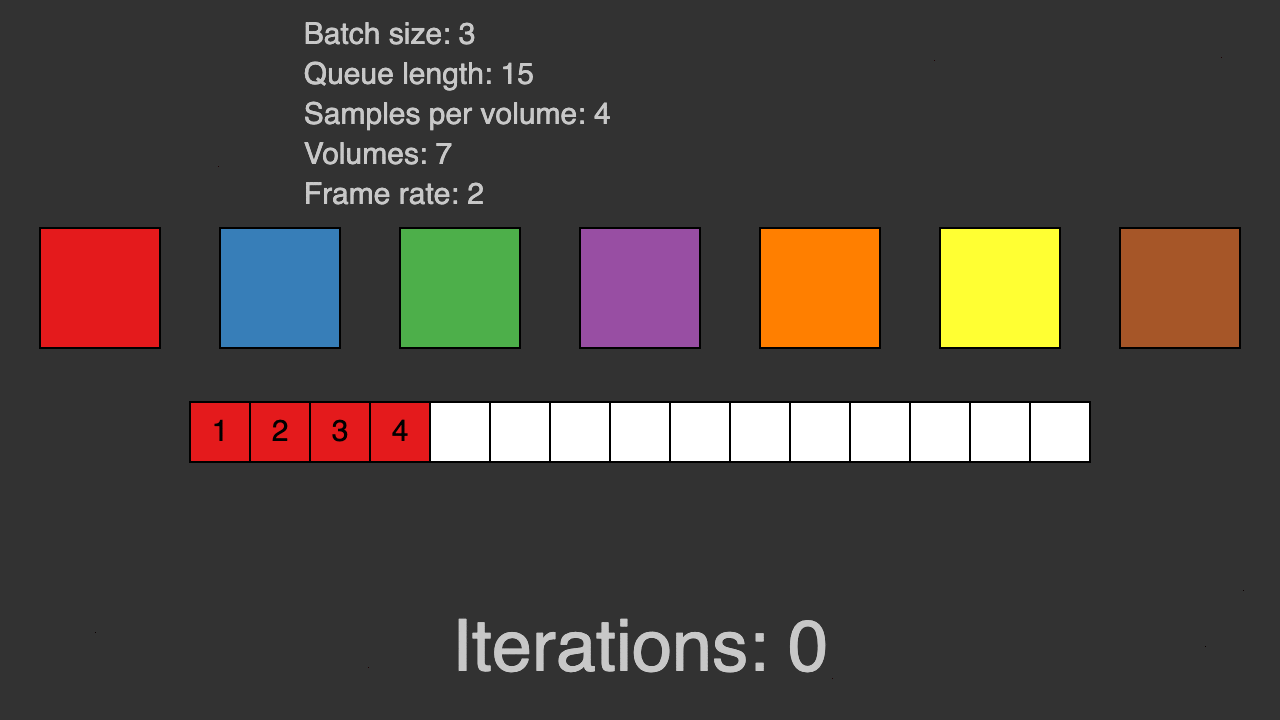
(Queue for patch-based training)
TorchIO is a Python package containing a set of tools to efficiently read, preprocess, sample, augment, and write 3D medical images in deep learning applications written in PyTorch, including intensity and spatial transforms for data augmentation and preprocessing. Transforms include typical computer vision operations such as random affine transformations and also domain-specific ones such as simulation of intensity artifacts due to MRI magnetic field inhomogeneity or k-space motion artifacts.
This package has been greatly inspired by NiftyNet, which is not actively maintained anymore.
If you like this repository, please click on Star!
If you use this package for your research, please cite the paper:
BibTeX entry:
@misc{fern2020torchio,
title={TorchIO: a Python library for efficient loading, preprocessing, augmentation and patch-based sampling of medical images in deep learning},
author={Fernando Pérez-García and Rachel Sparks and Sebastien Ourselin},
year={2020},
eprint={2003.04696},
archivePrefix={arXiv},
primaryClass={eess.IV}
}
See Getting started for installation instructions, a Hello, World! example and a comprehensive Jupyter notebook tutorial on Google Colab.
All the documentation is hosted on Read the Docs.
Please open a new issue if you think something is missing.
Thanks goes to all these people (emoji key):
Fernando Pérez-García 💻 📖 |
valabregue 🤔 👀 💻 |
GFabien 💻 |
G.Reguig 💻 |
Niels Schurink 💻 |
Ibrahim Hadzic 🐛 |
ReubenDo 🤔 |
Julian Klug 🤔 |
David Völgyes 🤔 |
Jean-Christophe Fillion-Robin 📖 |
Suraj Pai 🤔 |
Ben Darwin 🤔 |
This project follows the all-contributors specification. Contributions of any kind welcome!