Unpaired Image-to-Image Translation using Cycle-Consistent Adversarial Networks
Check the requirements.txt
- replay buffer
It will automatically download the data in data.py
.
python3 train.py
GAN-like networks are particularly challenging given that they often use multiple optimizers. In addition, GANs also consume a large amont of GPU memory and are usually batch-size sensitive.
To speed up training, we thus use a novel KungFu distributed training library. KungFu is easy to install and run (compared to today's Horovod library which depends on OpenMPI). You can install it using a few lines by following the instruction. KungFu is also very fast and scalable, compared to Horovod and parameter servers, making it an attractive option for GAN networks.
In the following, we assume that you have added kungfu-run
into the $PATH
.
(i) To run on a machine with 4 GPUs:
kungfu-run -np 4 python3 train.py --parallel --kf-optimizer=sma
The default KungFu optimizer is sma
which implements synchronous model averaging.
The sma
decouple batch size and the number of GPUs, making it hyper-parameter-robust during scaling.
You can also use other KungFu optimizers: sync-sgd
(which is the same as the DistributedOptimizer in Horovod)
and async-sgd
if you train your model in a cluster that has limited bandwidth and straggelers.
(ii) To run on 2 machines (which have the nic eth0
with IPs as 192.168.0.1
and 192.168.0.2
):
kungfu-run -np 8 -H 192.168.0.1:4,192.168.0.1:4 -nic eth0 python3 train.py --parallel --kf-optimizer=sma
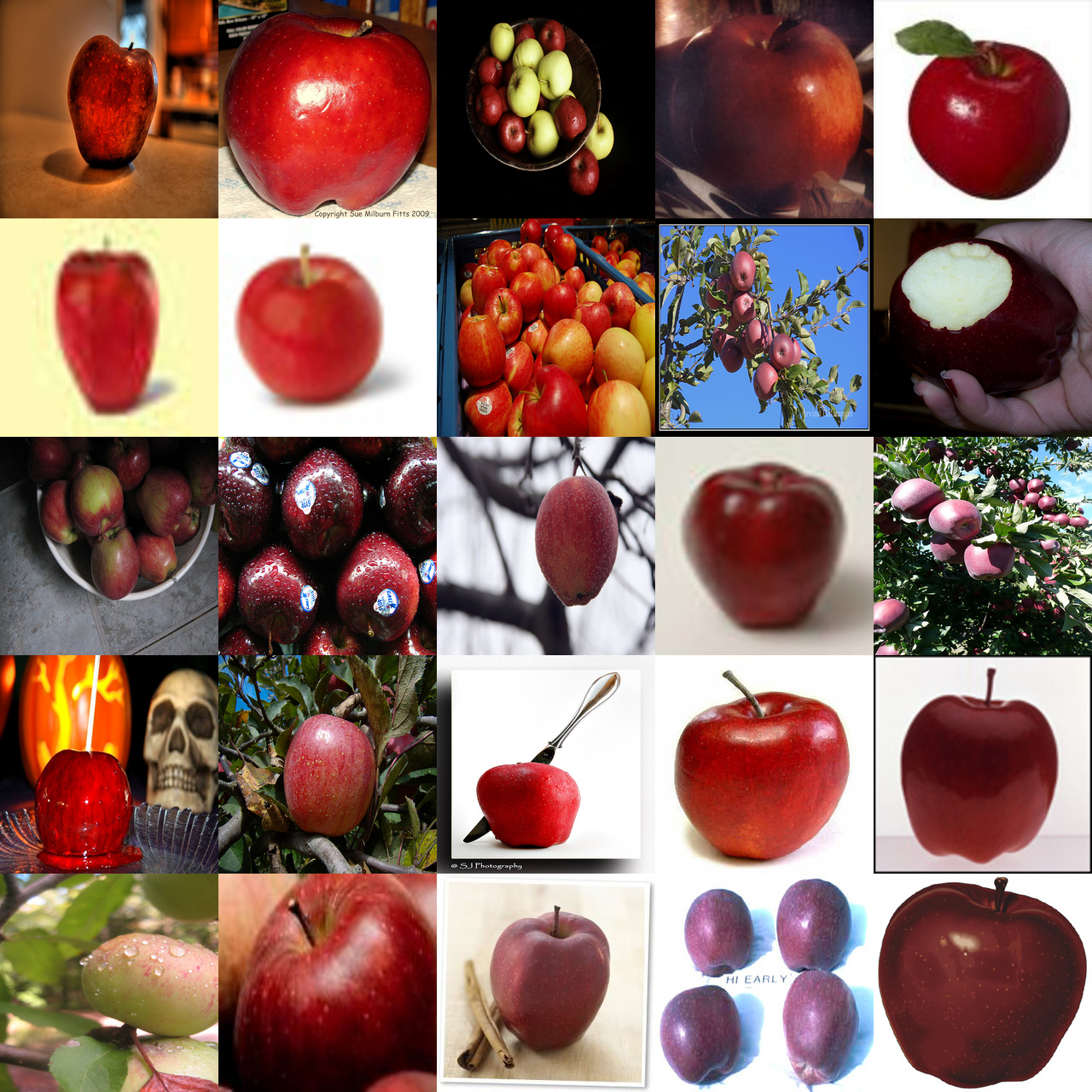
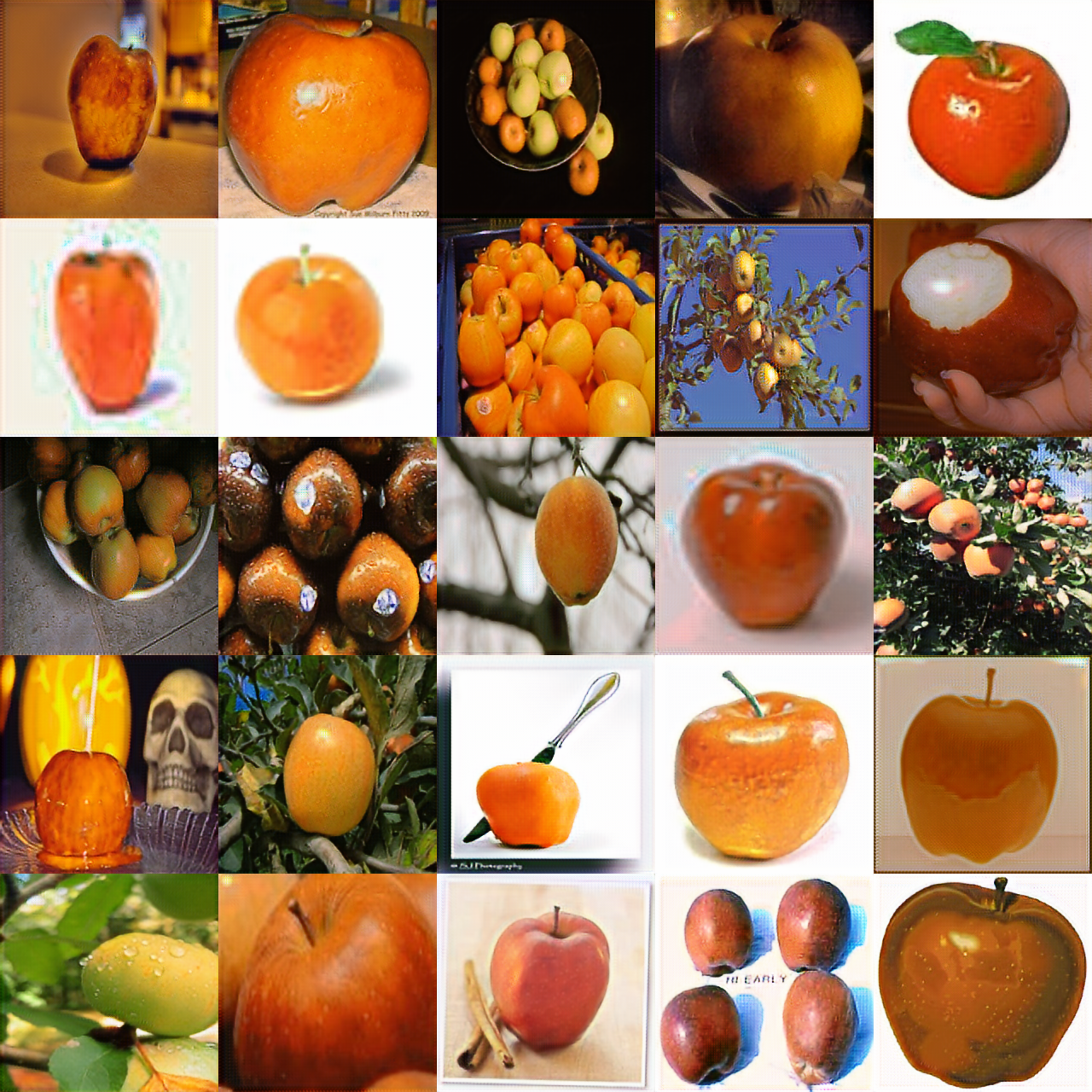
- @zsdonghao
- @luomai
- For academic and non-commercial use only.
- For commercial use, please contact tensorlayer@gmail.com.