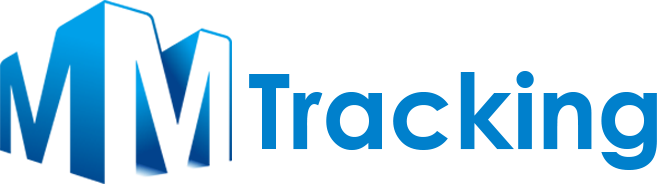
📘Documentation | 🛠️Installation | 👀Model Zoo | 🆕Update News | 🤔Reporting Issues
English | 简体中文
MMTracking is an open source video perception toolbox by PyTorch. It is a part of OpenMMLab project.
The master branch works with PyTorch1.5+.
-
The First Unified Video Perception Platform
We are the first open source toolbox that unifies versatile video perception tasks include video object detection, multiple object tracking, single object tracking and video instance segmentation.
-
Modular Design
We decompose the video perception framework into different components and one can easily construct a customized method by combining different modules.
-
Simple, Fast and Strong
Simple: MMTracking interacts with other OpenMMLab projects. It is built upon MMDetection that we can capitalize any detector only through modifying the configs.
Fast: All operations run on GPUs. The training and inference speeds are faster than or comparable to other implementations.
Strong: We reproduce state-of-the-art models and some of them even outperform the official implementations.
Release QDTrack pretrained models.
v0.13.0 was released in 29/04/2022. Please refer to changelog.md for details and release history.
Please refer to install.md for install instructions.
Please see dataset.md and quick_run.md for the basic usage of MMTracking.
A Colab tutorial is provided. You may preview the notebook here or directly run it on Colab.
There are also usage tutorials, such as learning about configs, an example about detailed description of vid config, an example about detailed description of mot config, an example about detailed description of sot config, customizing dataset, customizing data pipeline, customizing vid model, customizing mot model, customizing sot model, customizing runtime settings and useful tools.
Results and models are available in the model zoo.
Supported Methods
- DFF (CVPR 2017)
- FGFA (ICCV 2017)
- SELSA (ICCV 2019)
- Temporal RoI Align (AAAI 2021)
Supported Datasets
Supported Methods
- SiameseRPN++ (CVPR 2019)
- STARK (ICCV 2021)
- PrDiMP (CVPR2020) (WIP)
Supported Datasets
Supported Methods
- SORT/DeepSORT (ICIP 2016/2017)
- Tracktor (ICCV 2019)
- QDTrack (CVPR 2021)
- ByteTrack (arXiv 2021)
- OC-SORT (arXiv 2022) (WIP)
Supported Datasets
Supported Methods
- MaskTrack R-CNN (ICCV 2019)
Supported Datasets
We appreciate all contributions to improve MMTracking. Please refer to CONTRIBUTING.md for the contributing guideline and this discussion for development roadmap.
MMTracking is an open source project that welcome any contribution and feedback. We wish that the toolbox and benchmark could serve the growing research community by providing a flexible as well as standardized toolkit to reimplement existing methods and develop their own new video perception methods.
If you find this project useful in your research, please consider cite:
@misc{mmtrack2020,
title={{MMTracking: OpenMMLab} video perception toolbox and benchmark},
author={MMTracking Contributors},
howpublished = {\url{https://github.com/open-mmlab/mmtracking}},
year={2020}
}
This project is released under the Apache 2.0 license.
- MMCV: OpenMMLab foundational library for computer vision.
- MIM: MIM installs OpenMMLab packages.
- MMClassification: OpenMMLab image classification toolbox and benchmark.
- MMDetection: OpenMMLab detection toolbox and benchmark.
- MMDetection3D: OpenMMLab's next-generation platform for general 3D object detection.
- MMRotate: OpenMMLab rotated object detection toolbox and benchmark.
- MMSegmentation: OpenMMLab semantic segmentation toolbox and benchmark.
- MMOCR: OpenMMLab text detection, recognition and understanding toolbox.
- MMPose: OpenMMLab pose estimation toolbox and benchmark.
- MMHuman3D: OpenMMLab 3D human parametric model toolbox and benchmark.
- MMSelfSup: OpenMMLab self-supervised learning Toolbox and Benchmark.
- MMRazor: OpenMMLab Model Compression Toolbox and Benchmark.
- MMFewShot: OpenMMLab FewShot Learning Toolbox and Benchmark.
- MMAction2: OpenMMLab's next-generation action understanding toolbox and benchmark.
- MMTracking: OpenMMLab video perception toolbox and benchmark.
- MMFlow: OpenMMLab optical flow toolbox and benchmark.
- MMEditing: OpenMMLab image and video editing toolbox.
- MMGeneration: OpenMMLab Generative Model toolbox and benchmark.
- MMDeploy: OpenMMlab deep learning model deployment toolset.